Unlocking the Future: The Role of Malware Detection in Machine Learning
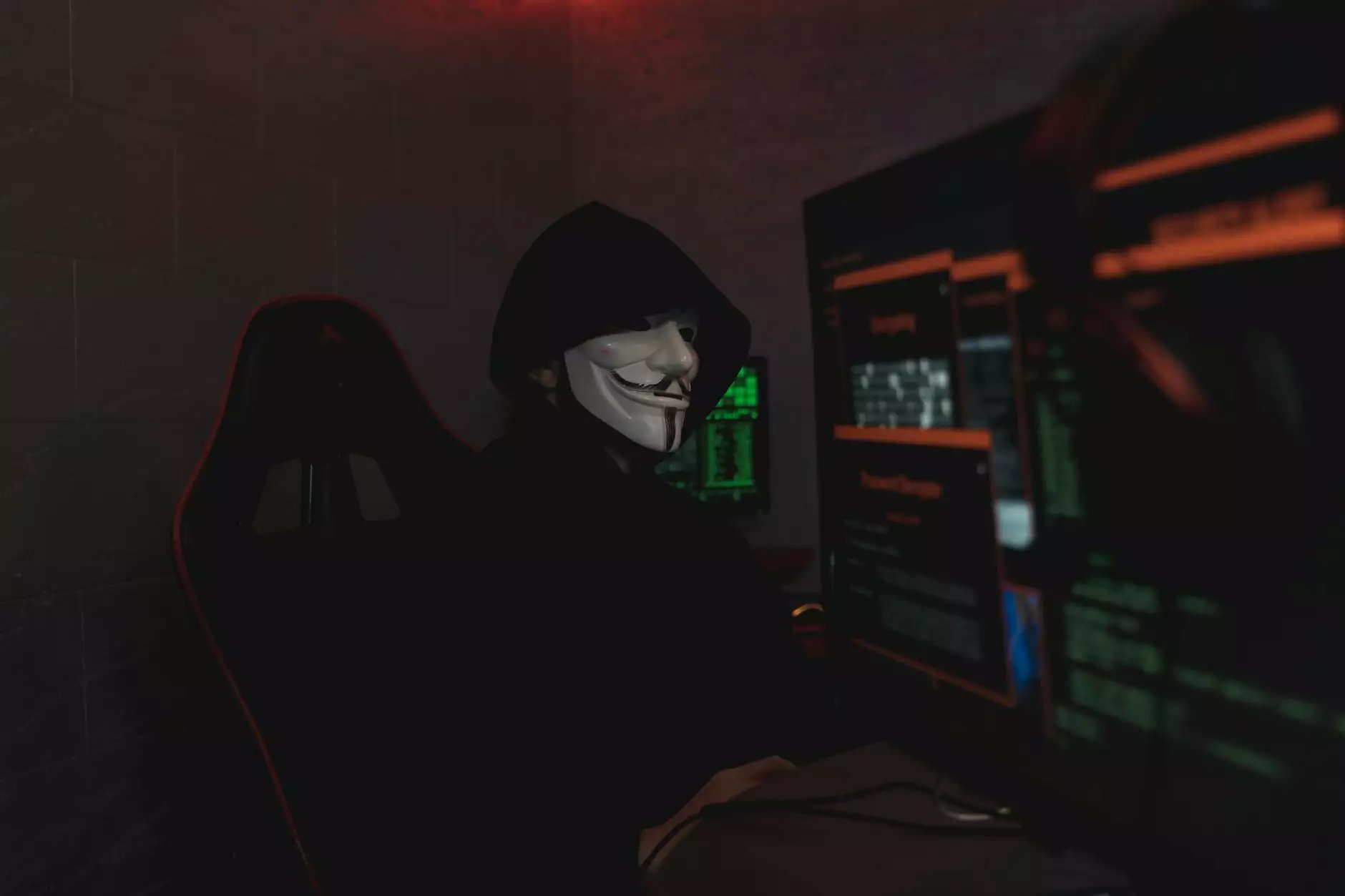
Introduction to Malware Detection and Machine Learning
In today’s digital age, the threat of malware has escalated to unprecedented levels. Organizations are increasingly relying on sophisticated methods to enhance their cybersecurity measures. One of the most revolutionary approaches in this domain is the integration of machine learning in malware detection. This article delves into the intricacies of how machine learning is transforming malware detection and providing unparalleled security solutions.
Understanding Malware and Its Impact
Malware, short for malicious software, includes a wide range of harmful programs designed to infiltrate, damage, or disable computers and networks. Different types of malware include:
- Viruses: Programs that replicate and spread to other devices.
- Worms: Standalone software that replicates itself across networks.
- Trojans: Malicious software disguised as legitimate software.
- Ransomware: Malware that locks or encrypts files until a ransom is paid.
- Spyware: Software that secretly gathers user information.
The impact of malware on businesses can be catastrophic, ranging from financial losses and compromised sensitive data to a tarnished reputation.
Traditional Methods of Malware Detection
In the past, malware detection primarily relied on signature-based detection methods. This approach compares files against a database of known malware signatures. While effective for detecting established threats, signature-based methods struggle with:
- Zero-Day Attacks: New malware that does not yet have a signature.
- Polymorphic Malware: Malware that changes its signature to evade detection.
As cyber threats evolve, there's a growing need for more advanced detection methods that can adapt to emerging threats.
Introducing Machine Learning in Malware Detection
Machine learning revolutionizes the way malware detection systems operate. By leveraging algorithms that learn from data, machine learning can identify patterns and anomalies indicative of malware, making it a powerful ally in cybersecurity.
Machine learning models can analyze vast amounts of data, recognizing both benign and malicious behaviors without relying solely on pre-existing signatures. This capability enables organizations to proactively defend against threats.
How Machine Learning Enhances Malware Detection
1. Behavioral Analysis
One of the core strengths of machine learning in malware detection is its ability to conduct behavioral analysis. Rather than merely identifying known malware, it assesses the behavior of applications to determine if they exhibit malicious patterns.
2. Anomaly Detection
Machine learning models can establish a baseline of normal activity within a system. Any deviation from this norm is flagged for investigation, allowing for the early detection of potential threats.
3. Continuous Learning
Machine learning algorithms continuously adapt and improve as they process more data. This means they become more effective over time, enhancing the security posture of organizations markedly.
4. Reduced False Positives
Traditional malware detection methods suffer from a high rate of false positives. Machine learning reduces this issue by accurately identifying genuine threats while minimizing unnecessary alerts.
Case Studies: Success Stories in Utilizing Machine Learning for Malware Detection
Numerous organizations have successfully implemented machine learning-based malware detection systems, leading to enhanced cybersecurity measures. Let's explore a few notable examples:
Case Study 1: Large Financial Institution
A prominent bank adopted a machine learning platform to monitor their network traffic. The system successfully detected a zero-day exploit that was previously undetected by traditional antivirus programs, safeguarding sensitive customer information.
Case Study 2: E-Commerce Company
An e-commerce giant implemented an anomaly detection system powered by machine learning to monitor transactions. This approach significantly decreased fraudulent activities, adding a layer of trustworthiness to their platform.
Challenges of Implementing Machine Learning in Malware Detection
While the benefits of machine learning in malware detection are clear, several challenges can impede its effective implementation:
- Data Privacy Concerns: Analyzing user behavior may raise privacy issues.
- Training Data Requirements: Machine learning models require large, diverse datasets for training, which can be hard to obtain.
- Complexity of Implementation: The integration of these systems into existing infrastructure can be complicated and resource-intensive.
The Future of Malware Detection with Machine Learning
The future of malware detection with machine learning is promising. As technology progresses, machine learning algorithms will become increasingly sophisticated:
- Advanced Deep Learning Techniques: Utilizing neural networks and deep learning could enhance detection rates significantly.
- Collaborative Approaches: Organizations may share threat intelligence, leveraging collective knowledge in machine learning algorithms.
- Integration with Other Security Measures: Combining machine learning with other security technologies such as firewalls and intrusion detection systems will create a more cohesive defense strategy.
Conclusion
The paradigm shift brought about by machine learning in malware detection cannot be overstated. It offers a proactive approach, adapting to new threats and learning from previous encounters. As cyber threats become more sophisticated, the need for such advanced solutions becomes imperative. Organizations like Spambrella are at the forefront of adopting these technologies, ensuring robust security frameworks and effective IT services.
Embracing machine learning in cybersecurity is not just a trend; it is a necessity for businesses aiming to shield themselves from malware threats and unauthorized intrusions in an increasingly digital landscape.
malware detection machine learning