Unlocking the Power of Machine Learning with Image Annotation Tools
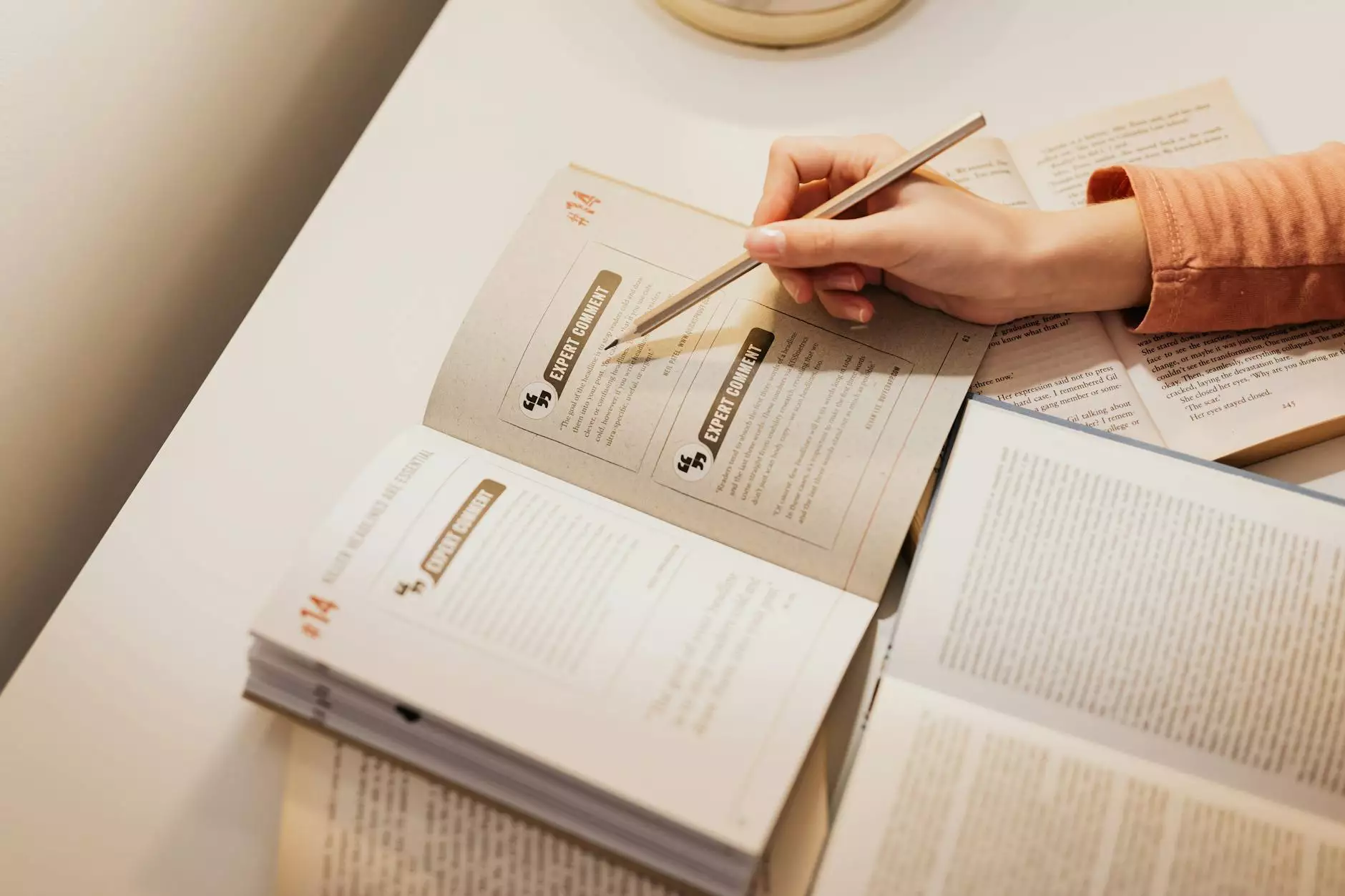
Introduction to Image Annotation in Machine Learning
In the realm of machine learning, the significance of data cannot be overstated. As algorithms become more sophisticated, the quality and quantity of data they are trained on directly impact their performance. One vital component of this data preparation process is image annotation. An effective image annotation tool for machine learning is essential for creating datasets that drive successful AI systems. This article will explore the benefits, applications, and features of image annotation tools, specifically tailored for machine learning.
What is Image Annotation?
Image annotation is the process of labeling and tagging images to provide context and meaning to raw data. It involves marking specific parts of an image, identifying objects, and describing attributes that allow machine learning models to learn from the data. The annotations can vary widely, from bounding boxes around objects to semantic segmentation, where each pixel is categorized.
Importance of Image Annotation for Machine Learning
The role of image annotation in machine learning is crucial for several reasons:
- Data Quality: Accurate and thorough annotations lead to higher quality datasets, which improve model performance.
- Training Efficiency: Well-annotated images help algorithms learn faster and more effectively, reducing time and computational resources.
- Model Accuracy: Precise image labels enhance the predictive capabilities of machine learning models, resulting in more effective solutions.
Types of Annotation Techniques
Various annotation techniques cater to different machine learning applications. Here are some widely utilized methods:
1. Bounding Box Annotation
This technique involves drawing rectangular boxes around objects of interest in an image. It's commonly used in applications such as object detection, where the location of items needs to be identified.
2. Semantic Segmentation
Semantic segmentation goes a step further by classifying each pixel in the image, thus providing more detailed information about the objects present. This technique is essential for applications like autonomous driving and medical imaging.
3. Instance Segmentation
Instance segmentation differentiates between individual objects of the same class. For example, in an image with multiple cars, instance segmentation can identify and differentiate each car as a distinct entity.
4. Keypoint Annotation
This approach marks specific points of interest, like joints in a human body or features in an object. It is particularly useful for pose estimation and facial recognition applications.
Choosing the Right Image Annotation Tool for Machine Learning
With numerous tools available, selecting the appropriate image annotation tool for machine learning can be daunting. Here are key factors to consider:
1. User Interface
A user-friendly interface allows annotators to work efficiently. Look for a tool that provides a seamless and intuitive experience.
2. Annotation Features
Different projects may require various annotation types. Ensure the tool supports the techniques you're interested in, such as bounding boxes, segmentation, or keypoint markers.
3. Scalability
As datasets grow, so must your annotation tool. Choose a tool that can scale alongside your projects, accommodating increasing amounts of data without sacrificing performance.
4. Collaboration Capabilities
In most cases, annotation is a team effort. Look for tools that facilitate collaboration, allowing multiple users to annotate and review tasks efficiently.
5. Integration with Machine Learning Frameworks
Opt for a tool that seamlessly integrates with popular machine learning frameworks to streamline the workflow from annotation to model training.
Benefits of Using an Image Annotation Tool
Utilizing a dedicated image annotation tool for machine learning offers numerous advantages:
1. Enhanced Accuracy
A specialized tool provides features that reduce errors and enhance labeling precision, contributing to better model training.
2. Increased Productivity
With built-in functionalities, annotators can work more efficiently, significantly reducing the time needed to prepare datasets.
3. Cost-Effectiveness
By improving efficiency and accuracy, businesses can save on costs associated with model retraining and deployment failures.
4. Customization Options
Many tools allow for customization to fit specific project needs, from creating custom labels to adjusting workflow processes.
Image Annotation in Various Industries
The applications of image annotation tools span multiple industries, each leveraging this technology to improve their operations:
1. Automotive Industry
In self-driving technology, precise annotations ensure that vehicles can accurately identify pedestrians, other vehicles, and road signs, enhancing overall safety on the roads.
2. Healthcare
In medical imaging, annotating images enables healthcare professionals to train algorithms for diagnosing conditions from X-rays, MRIs, and CT scans, facilitating faster and more accurate diagnoses.
3. Retail and E-commerce
Retailers use image annotation to analyze customer behavior through visual recognition of products, improving inventory management and enhancing user experience.
4. Agriculture
Farming applications utilize image annotation for crop monitoring and disease detection, contributing to increased productivity and sustainable practices.
Future Trends in Image Annotation for Machine Learning
As technology evolves, the field of image annotation is also progressing. Here are some emerging trends:
1. Automation in Annotation
Leveraging AI and machine learning to automate parts of the annotation process is gaining traction. This can significantly reduce time and human labor in data preparation.
2. Use of 3D Annotations
As industries move towards more complex visual datasets, 3D annotations are becoming essential, particularly in fields like gaming and virtual reality.
3. Enhanced Collaboration Tools
With remote work becoming the norm, collaborative annotation platforms that allow real-time teamwork will grow in importance.
4. Integration with Advanced Analytics
Future annotation tools will likely incorporate advanced analytics features to monitor progress, efficiency, and overall data quality in real-time.
Conclusion
In conclusion, the importance of a robust image annotation tool for machine learning cannot be overstated. As we advance into an era where data drives decision-making and innovation, investing in high-quality annotation processes is fundamental for the development of AI solutions that deliver accurate and reliable results. With the right tools, organizations can harness the full potential of their data, paving the way for advancements across multiple sectors.
Get Started with Keylabs.ai
If you are ready to explore high-performance image annotation solutions tailored for your needs, visit us at Keylabs.ai. Our Data Annotation Tool and Data Annotation Platform are designed to enhance your machine learning projects and improve data quality with precision and speed.