Effective Malware Analysis Using Machine Learning: Insights for Businesses
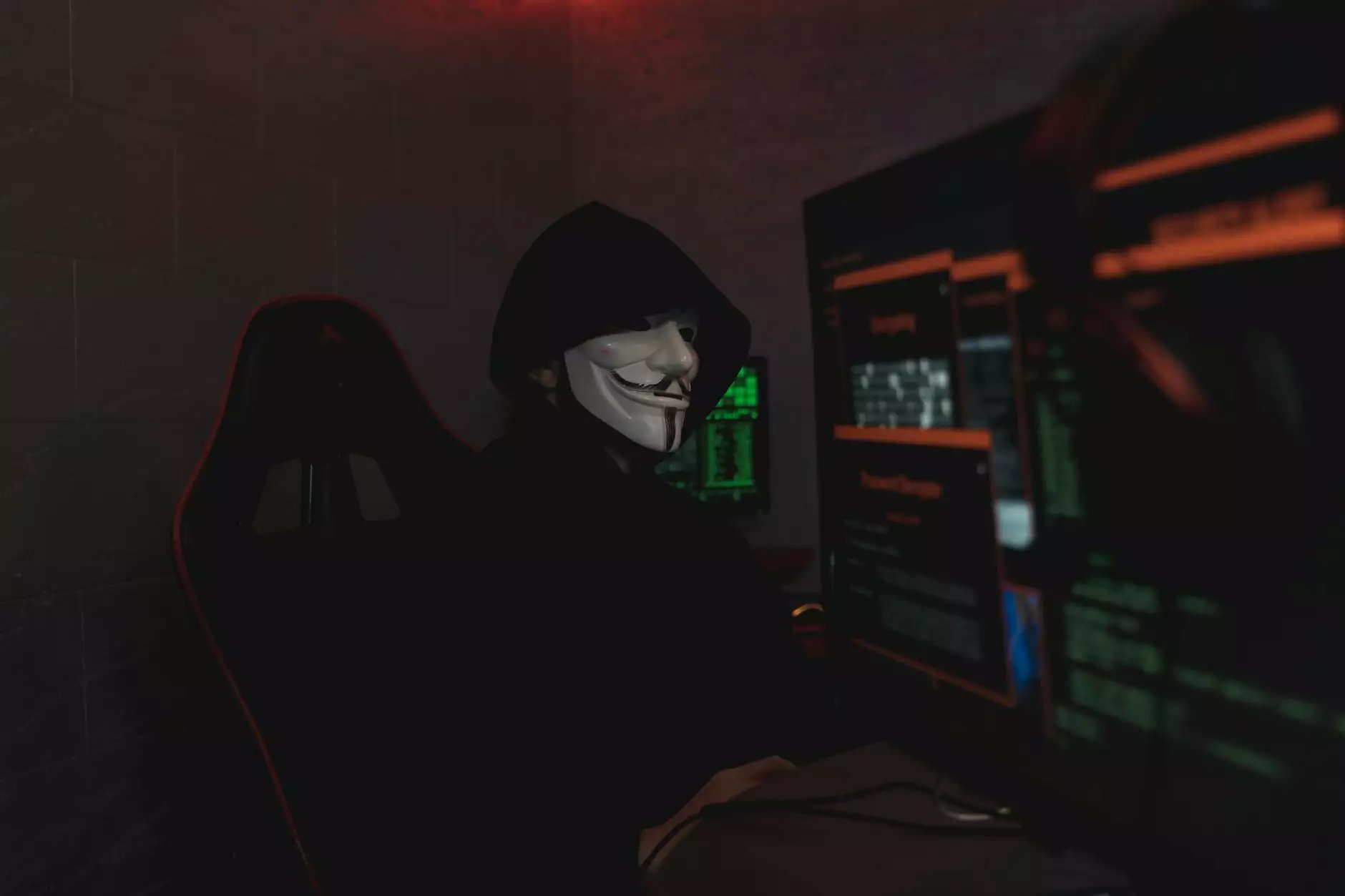
The digital landscape is fraught with challenges, especially when it comes to securing systems against malicious attacks. One of the most pressing concerns for businesses today is the growing frequency and complexity of malware threats. However, advancements in technology, particularly machine learning, are providing innovative solutions for robust malware analysis. In this comprehensive guide, we explore how businesses can effectively leverage malware analysis using machine learning to enhance their cybersecurity measures.
Understanding Malware and Its Impact on Businesses
Malware, short for malicious software, refers to any program or file that is harmful to a computer or network. Businesses face various types of malware, including viruses, worms, Trojans, ransomware, and spyware. Each type can have devastating effects, such as:
- Data Breaches: Unauthorized access to sensitive data can lead to significant financial loss and damage to reputation.
- Operational Disruption: Malware can paralyze operations, causing loss of productivity and revenue.
- Data Loss: Critical business data may be irretrievably lost due to malware attacks.
- Legal Consequences: Breaching data protection regulations can lead to hefty fines and legal action.
The Rise of Machine Learning in Cybersecurity
As malware evolves, so do the methods of detecting and neutralizing it. Machine learning (ML), a subset of artificial intelligence, has become a game-changer in the field of cybersecurity. By utilizing algorithms that enable computers to learn from data, businesses can significantly improve their ability to identify and mitigate malware threats. Here are key benefits of using machine learning in malware analysis:
- Automated Threat Detection: ML models can automatically analyze vast amounts of data, identifying patterns indicative of malware.
- Real-time Analysis: Immediate threat detection allows for faster response to potential attacks, minimizing damage.
- Adaptive Learning: ML systems can continuously learn from new data, adapting to emerging threats and changing techniques used by malicious actors.
- Enhanced Accuracy: With improved analysis methods, machine learning reduces false positives and negatives in malware detection.
Implementing Machine Learning for Malware Analysis
To take advantage of malware analysis using machine learning, businesses should consider implementing a structured approach. Here are the key steps to effectively integrate ML into a business's cybersecurity strategy:
1. Data Collection
The first step involves aggregating a comprehensive dataset that consists of known malware signatures, system logs, network traffic, and user behavior patterns. This data serves as the foundational training material for ML algorithms.
2. Feature Selection
Feature selection is critical in identifying the attributes that contribute most significantly to malware detection. This step involves choosing relevant data features that can enhance the model's performance.
3. Model Development
Utilizing various machine learning algorithms, such as supervised learning (decision trees, support vector machines) and unsupervised learning (clustering techniques), businesses can develop a model tailored for malware detection.
4. Training the Model
Training the ML model involves feeding it with the previously collected data, allowing it to learn the characteristics of malware and distinguish it from legitimate software.
5. Testing and Validation
Once the model is trained, it is essential to validate its effectiveness using a separate dataset. This step ensures that the model accurately identifies malware without significant errors.
6. Deployment and Monitoring
Deploying the machine learning model into the business’s existing cybersecurity infrastructure allows for continuous monitoring of system activity. Real-time alerts can help in addressing threats as they emerge.
Real-World Applications of Machine Learning in Malware Analysis
Several organizations have successfully implemented malware analysis using machine learning. Here are a few notable examples:
1. Predictive Analytics in Cybersecurity
Businesses use machine learning algorithms to predict future malware attacks based on historical data. This proactive approach allows organizations to preemptively reinforce their systems against anticipated threats.
2. Anomaly Detection
ML algorithms can identify abnormal behavior within network activity. For instance, significant deviations in data transmission can trigger alerts about potential malware infections, enabling swift investigation and response.
3. Email Security Solutions
Machine learning enhances email security by filtering out phishing attempts and suspicious attachments. Businesses can train ML models to recognize patterns typical of malware-laden emails, thus protecting their employees and systems.
Challenges in Malware Analysis Using Machine Learning
Despite its numerous advantages, malware analysis using machine learning presents certain challenges that businesses must navigate:
- Data Quality and Volume: The efficacy of machine learning models heavily relies on the quality and volume of data. Poor-quality data can lead to inaccurate predictions.
- Continuous Evolution of Malware: Malware developers continuously innovate, making it essential for ML models to also evolve. This ongoing development requires resources and expertise.
- Expertise Requirements: Implementing machine learning solutions necessitates skilled personnel who can develop, train, and maintain these models.
- Investment Costs: Initial setup and ongoing maintenance of machine learning systems can incur significant costs, which may be a concern for smaller businesses.
Conclusion: The Future of Malware Analysis in a Machine Learning Era
In the face of increasingly sophisticated cyber threats, malware analysis using machine learning serves as a vital component in enhancing business security. Companies like Spambrella are pioneering these developments, integrating advanced systems to safeguard against malware and protect sensitive information.
As businesses continue to digitize and incorporate technology into their operations, investing in machine learning for malware analysis is not just a trend; it's a necessity. By taking proactive measures and harnessing the power of machine learning, organizations can stay a step ahead of cyber adversaries, ensuring their systems remain secure and efficient.
Ultimately, understanding and applying malware analysis using machine learning is crucial for businesses that aim to thrive in an increasingly complex digital environment. With the right strategies and tools in place, organizations can protect their assets, maintain customer trust, and ensure uninterrupted operations.